Back in September, NVIDIA announced that it was set to acquire the chip designer giant ARM for a whopping $40 billion. This is kind of a big deal taking into account that ARM architecture is virtually everywhere these days, from top smartphone manufacturers such as Samsung and Apple utilizing it, to gaming consoles, home appliances, and various IoT devices that make use of a system-on-a-chip (SOC) design model. The business deal, currently under the processes of regulatory approval, is set to make ARM a subsidiary of NVIDIA while retaining its operational strategies and licensing partnerships, such as with Qualcomm, Samsung, Apple, and others.
Arguably, NVIDIA itself, as the leading supplier of GPUs to the PC industry, can be considered an AI company looking to cement itself as another catalyst in the transition of artificial intelligence to the edge. With over 500 customer corporations that are responsible for licensing ARM technology, NVIDIA has correctly recognized the real value of the chip vendor’s ecosystem — a worthy starting point to introduce the flow of AI technology to smart devices.
What does this mean for the Smartphone Market?
Of the main players in the GPU market for personal computers, NVIDIA holds a rather dominant position, with Intel and AMD being the other significant competitors in that sector. In contrast, ARM does not face any notable competition which could allow NVIDIA to establish a hold over the smartphone market. Devices powered with Arm CPU + Nvidia GPU technology could very well usher in a new generation of smartphone chipsets and hardware. While it doesn’t seem very likely that next year’s flagships come shipped with full blown GeForce RTX accelerators, it’s not too far-flung to assume that current ARM compatible GPUs might start embedding some form of tech derived from NVIDIA’s R&D. For a fact, Samsung has already partnered with AMD and based on some leaked stories, their 2021 flagship is set to include a custom Radeon graphics processor.
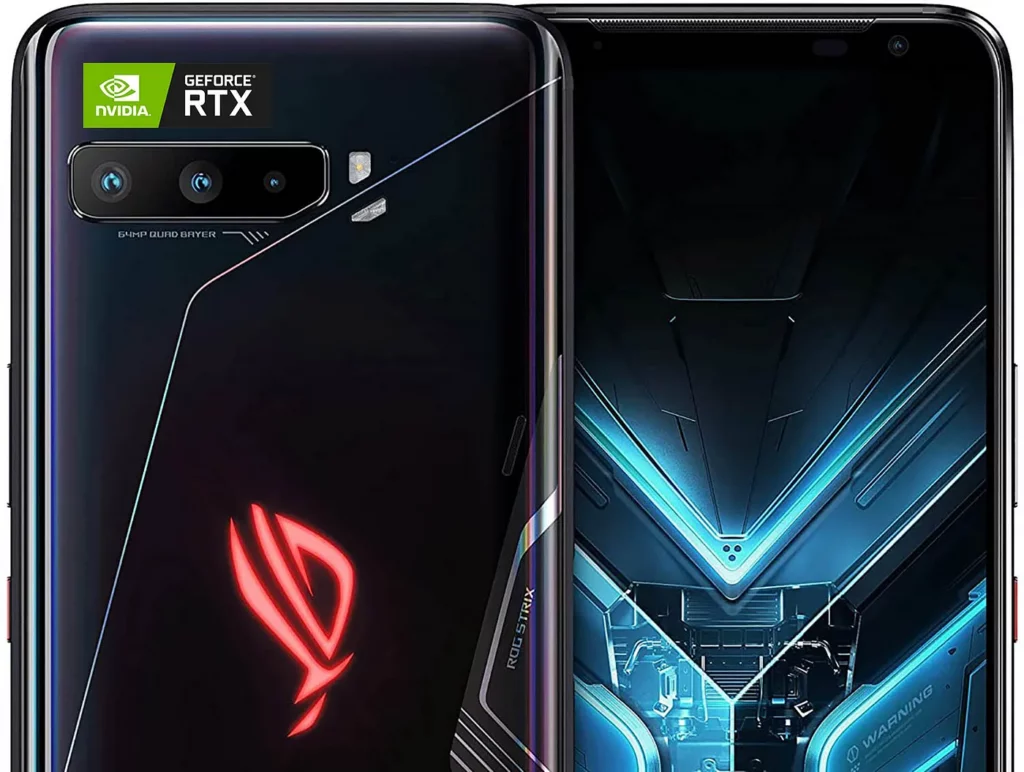
Not only does this mean that we could use our phones to play some games with truly amazing and high-end graphics, but we may even allow them to harness deep learning capabilities like never before, born out of the power of dedicated, powerful GPUs. NVIDIA has made it quite clear that it is looking to utilize ARM’s vast network of SOCs to integrate its AI technologies into products. According to CEO Jensen Huang,“our dream is to bring NVIDIA’s AI to ARM’s ecosystem, and the only way to bring it to the ARMs ecosystem is through all of the existing customers, licensees, and partners.”
The smartphone market is not the only one prepping to see some major changes. Apple recently announced their intention to transition from using traditional Intel processors in Macbooks to their own in-house manufactured chips that are based on ARM technology and similar to the A-series chips used in iPhones and iPads. Other events, such as the development of Windows on ARM processors, also stand to afford certain advantages to NVIDIA’s strategy.
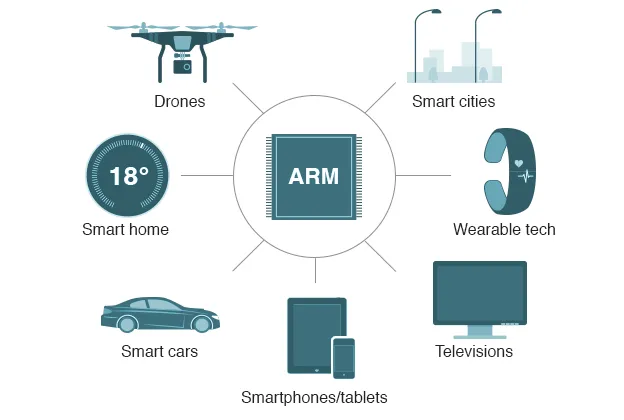
ARM + NVIDIA: A new age in mobile AI ?
NVIDIA is well established as one of the biggest go-to companies in the world of AI. For years now, most deep learning systems have been trained on NVIDIA GPUs and specialized hardware. Most cloud service providers who incorporate machine learning as a service also make use of NVIDIA accelerators in their data centers. NVIDIA has been focused on growing its market of AI services to the edge computing market as well. NVIDIA’s EGX AI platform is one such effort to “deliver the power of accelerated AI computing from data center to edge with a range of optimized hardware, an easy-to-deploy, cloud-native software stack and management service, and a vast ecosystem of partners who offer EGX through their products and services,” according to their webpage.
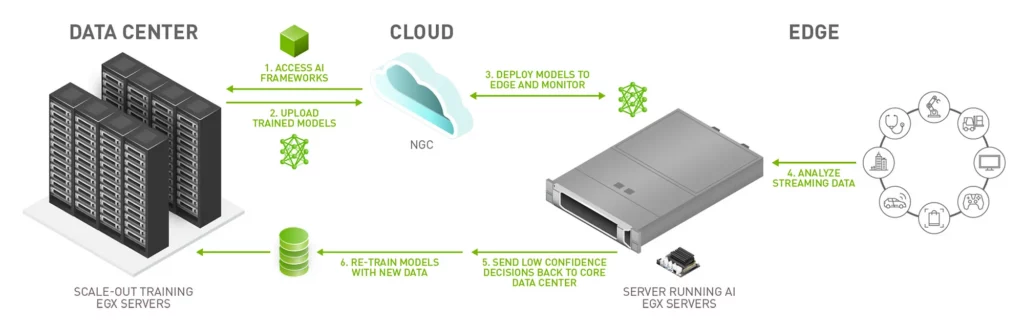
One of the challenges that oppose an integration of current dedicated GPU technology on mobile devices is that graphics accelerators, especially the ones used in powering AI-heavy applications, are very power-hungry, with the overall power consumption associated with training and running neural networks becoming a significant issue for devices such as smartphones. In contrast, ARM chipsets are designed particularly to be as power-efficient as possible. Doubtless, seeing how NVIDIA handles this particular challenge to bring better hardware to edge devices will soon be a highlight in many tech articles.
NVIDIA also announced their not-so-long-term goals for a market that is saturated with AI applications that run on NVIDIA hardware in a wide range of devices, including phones, edge networks, and cloud-based data centers.
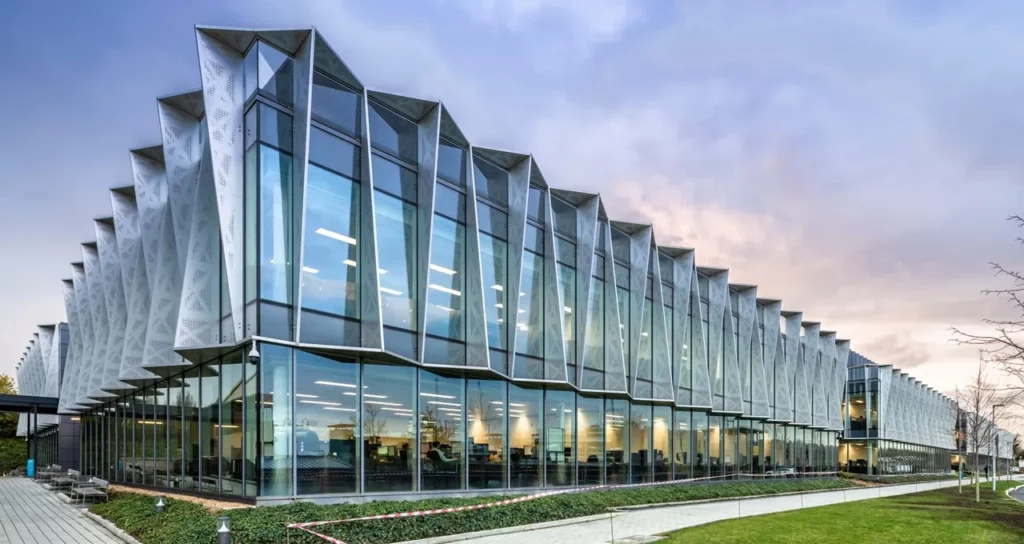
NVIDIA is also set to launch a world-class AI laboratory in Cambridge, U.K., at the ARM headquarters, dubbing it the Hadron collider/Hubble telescope for artificial intelligence. Many leading scientists, engineers, and researchers from around the world are expected to collaborate here and conduct work in areas such as healthcare, life sciences, self-driving cars, and other fields. The initiative is perhaps a counter to several critics claiming that outsourcing the predominantly British ARM to American company NVIDIA might not be in the U.K. economy’s best interests.
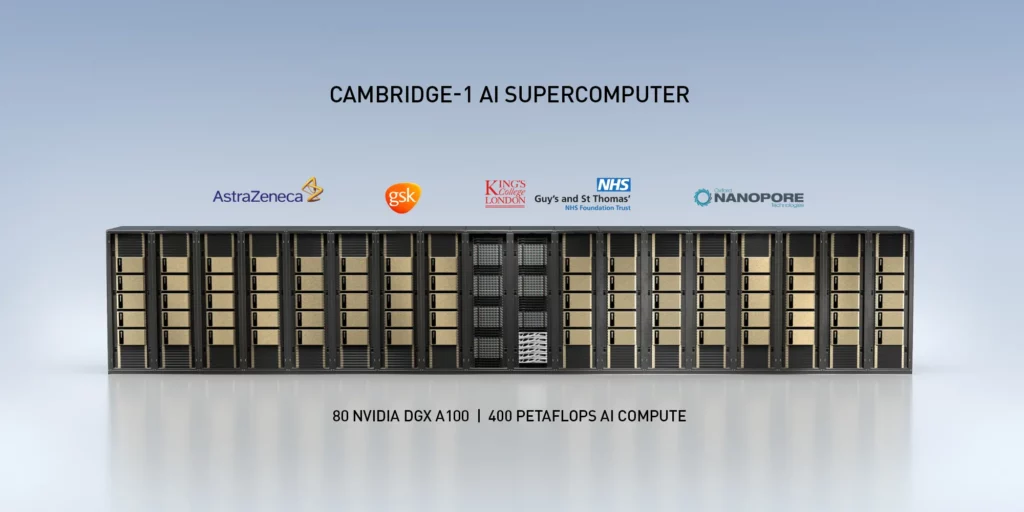
Additionally, NVIDIA announced that they will be undertaking the process of building Britain’s most powerful supercomputer to date, using ARM-based technology and hardware, which will use the most advanced AI yet to help researchers solve pressing medical challenges, including those related to COVID-19. Named Cambridge-1, the supercomputer, which is expected to come online by year end or early 2021 in Cambridge, will be a NVIDIA DGX SuperPOD system capable of delivering more than 400 petaflops of AI performance. Dedicated towards healthcare research efforts, GSK and AstraZeneca, which are both among the top contenders in the race for a coronavirus vaccine, will be two of the first pharmaceutical companies to harness the supercomputer’s power of AI.
Some other efforts that are to warrant the machine’s capabilities in the short term are:
Solving large-scale industrial data-science problems, which present an issue for conventional methods because of their size.
Access to NVIDIA GPU time which will be donated as a resource to local universities for specific research purposes. For example, Oxford’s quest for a COVID-19 cure.
Affording opportunities to learn and collaborate for AI start-ups to help them develop innovative changes.
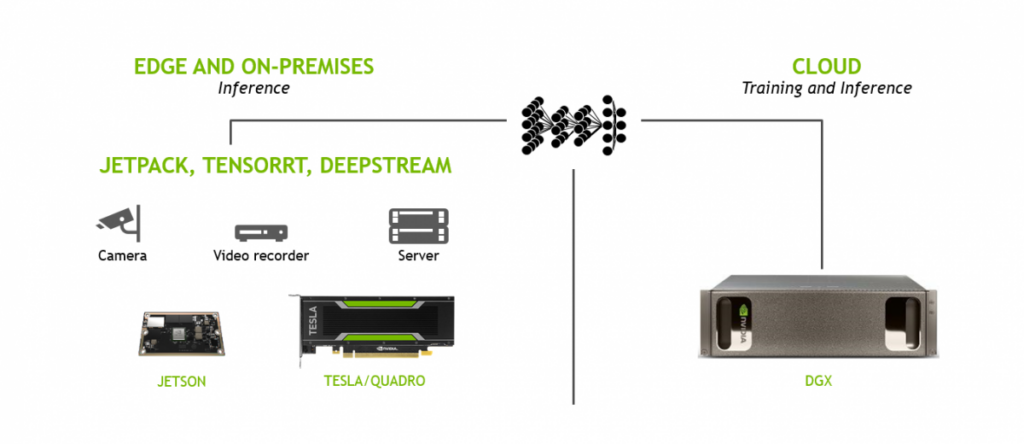
On-Device AI: A step closer?
With tech companies continuing to make the move towards on-device AI or “offline” AI for mobile and edge devices, it will be interesting to see how this development will make the case for better independent AI capabilities in ARM-based devices. NVIDIA is a known advocate for diversifying its artificial intelligence model into both cloud and on-premise dependencies. For instance, their release of the $99 pocket computer Jetson Nano last year, which is targeted towards “embedded designers, researchers, and DIY makers who want to tinker with a system for offline AI development.”
Competing with the likes of Google’s Edge TPU and Raspberry Pi, the SDK is powered by a 64-bit quad-core ARM processor, and a 128-core NVIDIA Maxwell GPU with 4GB of RAM to deliver processing speeds up to 472 Gflops. It hosts a bunch of ports like USB-A 3.0, HDMI, DisplayPort, and ethernet to plug in external hardware devices like camera modules and displays, and to connect to the internet.
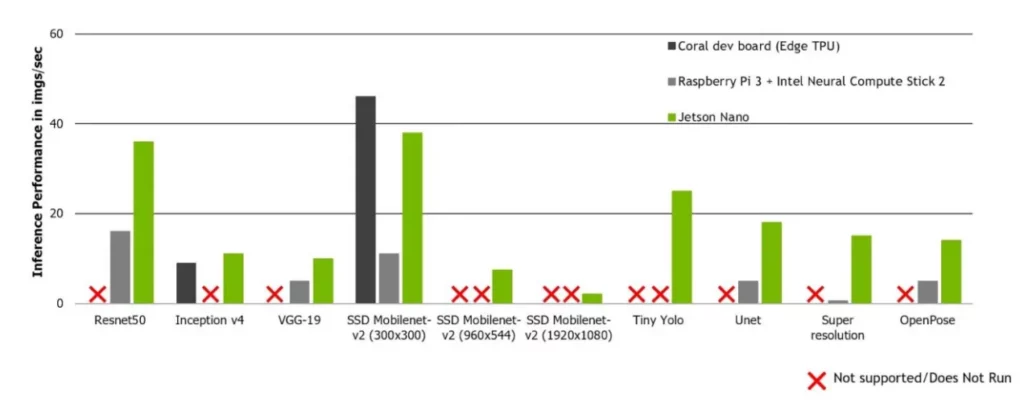
More recently, NVIDIA showcased the DGX Station A100, an “AI data-centre-in-a-box” powered by four 80GB Tensor Core GPUs. As a self-contained data center, the A100 provides a centralized AI resource for various AI workloads such as training, inference, data analytics, etc. Designed for today’s agile data science teams working in corporate offices, labs, research facilities, or even from home, DGX Station A100 requires no complicated installation or significant IT infrastructure. All you have to do is plug it into any standard wall outlet and it’ll be running within minutes, allowing you to work from anywhere. For organizations looking to utilize their intranet with little to no dependence on external networks, this presents an excellent choice. It is also a key element in NVIDIA HGX AI supercomputing platform, which is aimed at helping researchers and scientists to combine HPC, data analytics, and deep learning computing methods to advance scientific progress.
It seems in all probability that big changes are in order for the edge/mobile AI market as a derivative of the NVIDIA-ARM merger deal. We know that NVIDIA is a global leader in AI and has been pretty open in their intentions to leverage the ARM ecosystem and device network to transform the AI field for edge computing and smart devices. It remains only to be seen the magnitude and period of time it takes to implement the big changes quoted by them. From powerful GPUs on smartphones for boosting AI capabilities to smart cities featuring groundbreaking edge-to-cloud technologies, let’s wait and watch as NVIDIA brings their visions to reality.
Comments 0 Responses